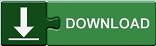
This service has been used to label the reports in AudGenDB and is freely available.Įlectronic health records (EHRs) contain significant amounts of unstructured text that pose a challenge to their secondary use as a research data source. We additionally implemented the models as a web service that accepts radiology report text in an HTTP request and provides the predicted region labels.
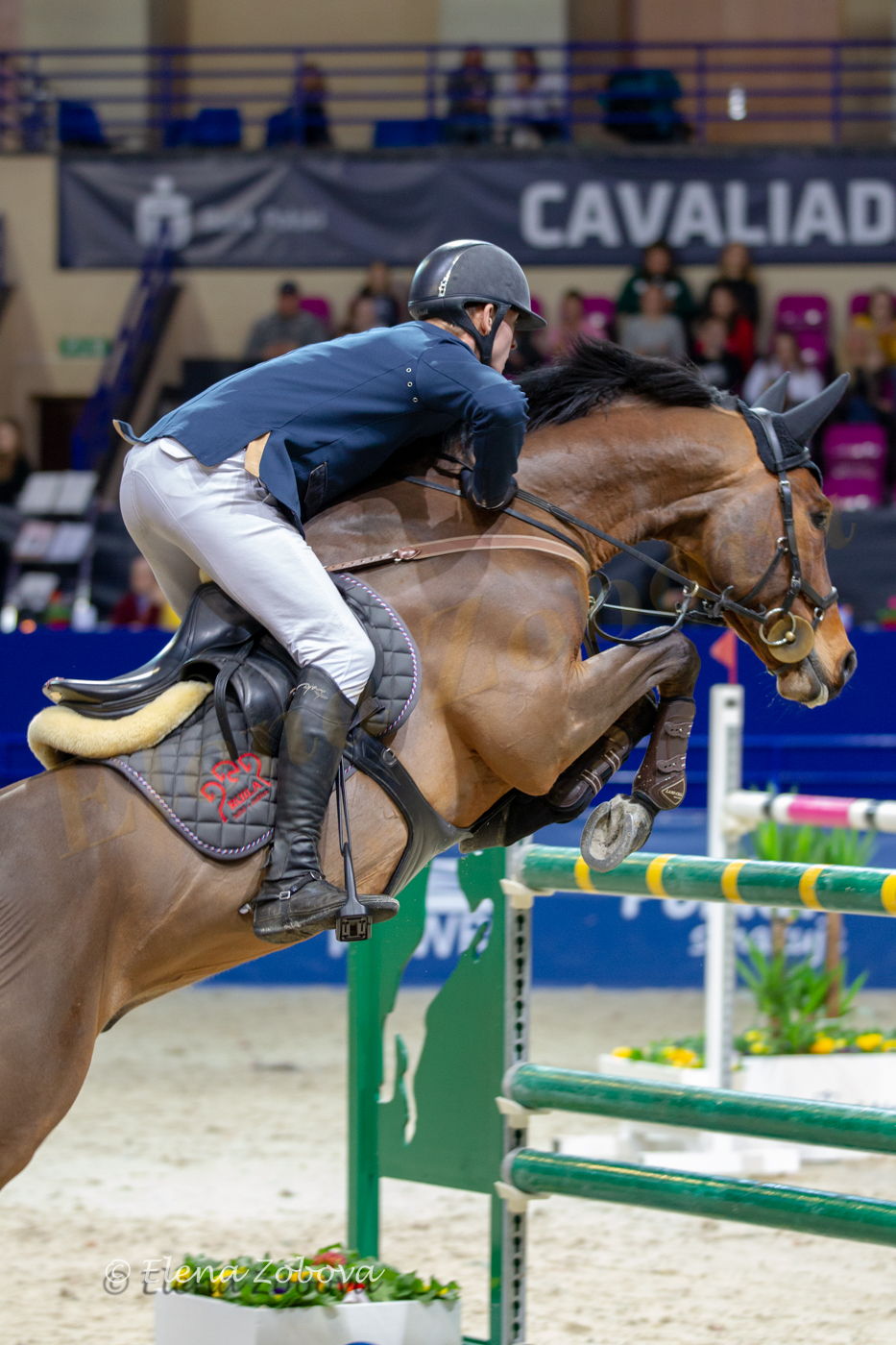
The models described here are available in several free, open source libraries that make them more accessible and simplify their utilization as demonstrated in this work. Our results indicate that the applied methods achieve accuracy scores sufficient to support our objective of extracting discrete features from radiology reports to enhance cohort identification in AudGenDB. The logistic regression method was very consistent, achieving accuracy scores within 2.75 % of the best classifier across regions and a receiver operator characteristic area under the curve of 0.92 or greater across all regions. Classifier test set accuracy was 90 %, 90 %, 93 %, and 82 % for the inner, middle, outer and mastoid regions, respectively. The best classifiers were linear support vector machine for inner and outer ear, logistic regression for middle ear, and decision tree for mastoid. Our gold-standard data set contained 726 reports. The models were evaluated on the hold-out test set. We trained logistic regression, support vector machine (linear and Gaussian), decision tree, random forest, and naïve Bayes models for each ear region. We applied open source libraries to normalize and convert every report to an n-gram feature vector. These were split into training (80 %) and test (20 %) sets. Trained abstractors labeled radiology reports taken from AudGenDB to form a gold standard. We implemented a classification pipeline using a human-in-the-loop machine learning approach and open source libraries to label the reports with one or more of four abnormality region labels: inner, middle, outer, and mastoid, indicating the presence of an abnormality in the specified ear region. Because the reports are unlabeled, it is difficult to select those with specific abnormalities. The Audiological and Genetic Database (AudGenDB) is a public, de-identified research database that contains over 16,000 radiology reports. Prior to utilization, trained experts must manually review reports to identify discrete outcomes. Kullo has authored 248 scientific papers, and he is the co-Principal Investigator of the Mayo electronic Medical Records and GEnomics (eMERGE) grant.Radiology reports are a rich resource for biomedical research.
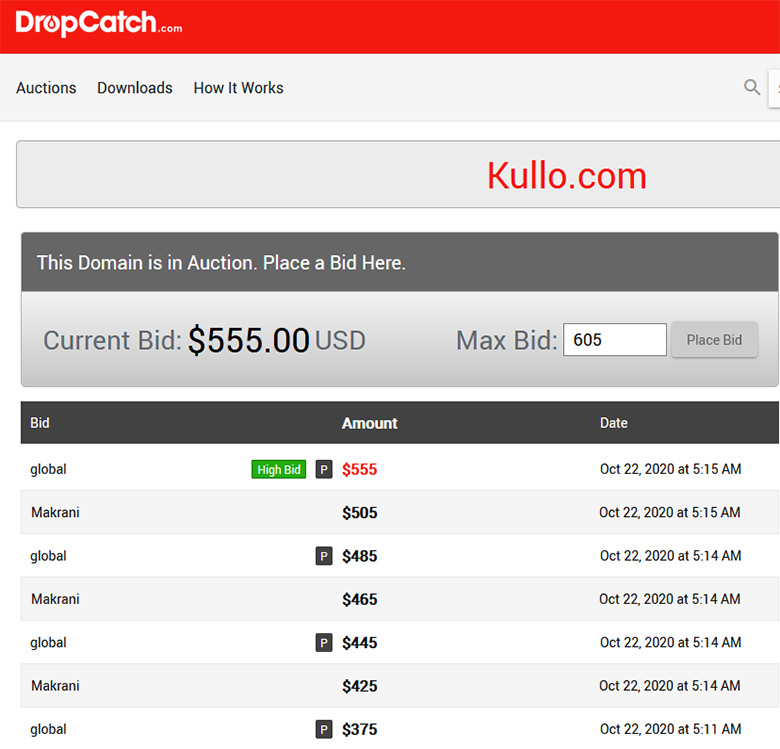
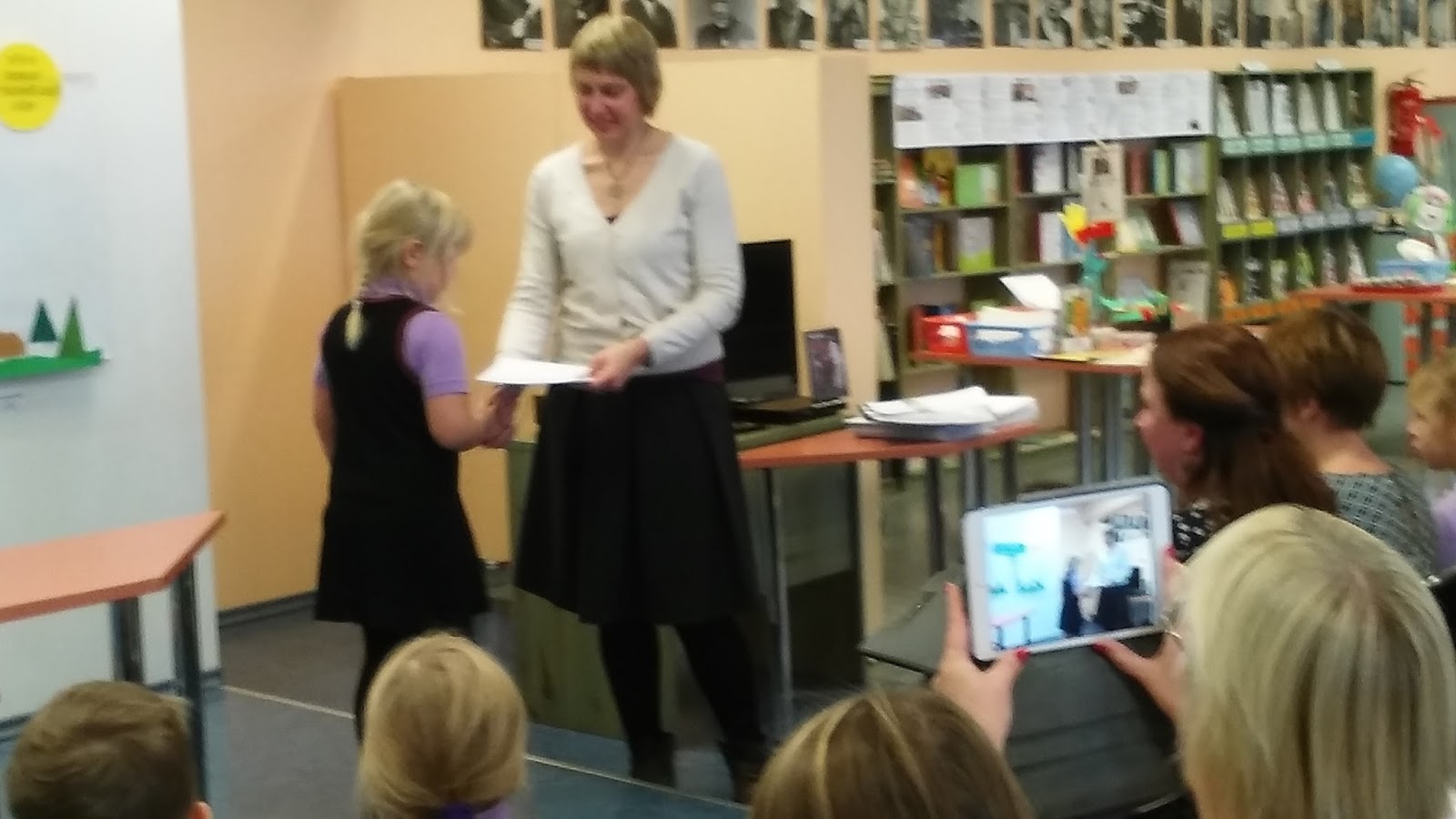
He directs the Early Atherosclerosis and Familial Hypercholesterolemia Clinics at Mayo Clinic Rochester, these evaluate and treat patients with premature vascular disease and genetic dyslipidemias.ĭr.
Csillag kullo trial#
Kullo led the MI-GENES study - the first genomic medicine clinical trial to demonstrate that incorporating genetic risk information can alter a health outcome. His research program is funded by the NIH since 2003 and includes both genomic medicine discovery and implementation projects.Kullo's research is focused on the genetics of atherosclerosis and lipid disorders. He completed residency training in Internal Medicine, including the Clinician Investigator training pathway and subsequently a Fellowship in Cardiovascular Diseases at Mayo Clinic. Iftikhar Kullo is a Professor of Medicine at Mayo Clinic Rochester, MN.
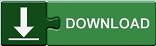